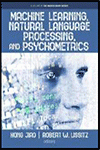
Machine Learning, Natural Language Processing, and Psychometrics
Edited by:
Hong Jiao, University of Maryland
Robert W. Lissitz, University of Maryland
A volume in the series: The MARCES Book Series. Editor(s): Hong Jiao, University of Maryland. Robert W. Lissitz, University of Maryland.
In Press 2024
With the exponential increase of digital assessment, different types of data in addition to item responses become available in the measurement process. One of the salient features in digital assessment is that process data can be easily collected. This non-conventional structured or unstructured data source may bring new perspectives to better understand the assessment products or accuracy and the process how an item product was attained. The analysis of the conventional and non-conventional assessment data calls for more methodology other than the latent trait modeling.
Natural language processing (NLP) methods and machine learning algorithms have been successfully applied in automated scoring. It has been explored in providing diagnostic feedback to test-takers in writing assessment. Recently, machine learning algorithms have been explored for cheating detection and cognitive diagnosis. When the measurement field promote the use of assessment data to provide feedback to improve teaching and learning, it is the right time to explore new methodology and explore the value added from other data sources. This book presents the use cases of machine learning and NLP in improving the assessment theory and practices in high-stakes summative assessment, learning, and instruction. More specifically, experts from the field addressed the topics related to automated item generations, automated scoring, automated feedback in writing, explainability of automated scoring, equating, cheating and alarming response detection, adaptive testing, and applications in science assessment. This book demonstrates the utility of machine learning and NLP in assessment design and psychometric analysis.
CONTENTS
The Item Factory: Intelligent Automation in Support of Test Development at Scale, Alina A. von Davier, Andrew Runge, Yena Park, Yigal Attali, Jacqueline Church, and Geoff LaFlair. Applications of Transformer Neural Networks in Processing Examinee Responses, Susan Lottridge. Integrating Natural Language Processing for Writing Assessment: Writing Trait Model, Paul Deane and Duanli Yan. Empirical Ensemble Equating Under the NEAT Design Inspired by Machine Learning Ideology, Zhehan Jiang, Yuting Han, Jihong Zhang, Lingling Xu, Dexin Shi, Haiying Liang, and Jinying Ouyang. Test Security in Remote Testing Age: Perspectives From Process Data Analytics and AI, Jiangang Hao and Michael Fauss. Using Language Models to Detect Alarming Student Responses, Chirstopher Ormerod, Milan Patel, and Harry Wang. EPIC Analysis: Evaluating Phrases in Context to Better Understand AI Scoring of Essays, Steven Tang. Fully Data-Driven Computerized Adaptive Testing, Aritra Ghosh, Wanyong Feng, Stephen Sireci, and Andrew Lan. From Adaptive Testing to Personalized Adaptive Testing: Applications of Recommender Systems, Okan Bulut. AI and Machine Learning for Next Generation Science Assessments, Xiaoming Zhai. An AI-Based Platform for Real Time Assessment, Scaffolding, and Alerting on Students’ Science Practices, Janice D. Gobert and the Inq-ITS Research & Development Team.
-
Paperback979-8-88730-604-9
Web price: $45.04 (Reg. 52.99)
-
Hardcover979-8-88730-605-6
Web price: $80.74 (Reg. 94.99)
- eBook979-8-88730-606-3
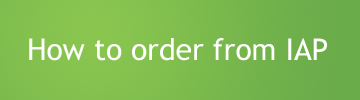
- COM042000 - PSYCHOLOGY: PSYCHOTHERAPY: Group: Artificial Intelligence: Natural Language Processing
- EDU039000 - EDUCATION: Computers & Technology
- TEC046000 - TECHNOLOGY & ENGINEERING: Machinery
-
Application of Artificial Intelligence to Assessment
-
Data Analytics and Psychometrics Informing Assessment Practices
-
Enhancing Effective Instruction and Learning Using Assessment Data
-
Innovative Psychometric Modeling and Methods
-
Technology Enhanced Innovative Assessment Development, Modeling, and Scoring From an Interdisciplinary Perspective
-
Test Fairness in the New Generation of Large‐Scale Assessment
-
The Next Generation of Testing Common Core Standards, Smarter‐Balanced, PARCC, and the Nationwide Testing Movement