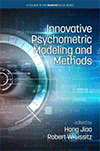
Innovative Psychometric Modeling and Methods
Edited by:
Hong Jiao, University of Maryland
Robert W. Lissitz, University of Maryland
A volume in the series: The MARCES Book Series. Editor(s): Hong Jiao, University of Maryland. Robert W. Lissitz, University of Maryland.
Published 2020
The general theme of this book is to present innovative psychometric modeling and methods. In particular, this book includes research and successful examples of modeling techniques for new data sources from digital assessments, such as eye-tracking data, hint uses, and process data from game-based assessments. In addition, innovative psychometric modeling approaches, such as graphical models, item tree models, network analysis, and cognitive diagnostic models, are included.
Chapters 1, 2, 4 and 6 are about psychometric models and methods for learning analytics. The first two chapters focus on advanced cognitive diagnostic models for tracking learning and the improvement of attribute classification accuracy. Chapter 4 demonstrates the use of network analysis for learning analytics. Chapter 6 introduces the conjunctive root causes model for the understanding of prerequisite skills in learning. Chapters 3, 5, 8, 9 are about innovative psychometric techniques to model process data. Specifically, Chapters 3 and 5 illustrate the usage of generalized linear mixed effect models and item tree models to analyze eye-tracking data. Chapter 8 discusses the modeling approach of hint uses and response accuracy in learning environment. Chapter 9 demonstrates the identification of observable outcomes in the game-based assessments. Chapters 7 and 10 introduce innovative latent variable modeling approaches, including the graphical and generalized linear model approach and the dynamic modeling approach.
In summary, the book includes theoretical, methodological, and applied research and practices that serve as the foundation for future development. These chapters provide illustrations of efforts to model and analyze multiple data sources from digital assessments. When computer-based assessments are emerging and evolving, it is important that researchers can expand and improve the methods for modeling and analyzing new data sources. This book provides a useful resource to researchers who are interested in the development of psychometric methods to solve issues in this digital assessment age.
CONTENTS
Advances in Psychometric Methods for Uncovering Latent Structure and Cognitive Processes, Steven Andrew Culpepper. Improving Attribute Classification Accuracy in High Dimensional Data: A Four-Step Latent Regression Approach, Yan Sun and Jimmy de la Torre. A Dynamic Generalized Mixed Effect Model for Intensive Binary Temporal-Spatio Data From an Eye-Tracking Technique, Sun-Joo Cho, Sarah Brown-Schmidt, Matthew Naveiras, and Paul De Boeck. Application of Network Analysis in Understanding Collaborative Problem-Solving Processes and Skills, Mengxiao Zhu, Jessica Andrews-Todd, and Mo Zhang. IRTree Modeling of Cognitive Processes Based on Outcome and Intermediate Data, Paul De Boeck and Sun-Joo Cho. Prerequisite Structure Finding Using the Conjunctive Root Causes Model, Xinchu Zhao, Benjamin Deonovic, and Gunter Maris. A Graphical and Generalized Linear Model Approach to Latent Variable Modeling, Frank Rijmen. Modeling Hint Requests, Response Times, and Response Accuracy in Adaptive Learning Systems, Maria Bolsinova, Benjamin Deonovic, Meirav Attali, and Gunter Maris. Identifying Observable Outcomes in Game-Based Assessments, Russell Almond, Valerie J. Shute, Seyfullah Tingir, and Seyedahmad Rahimi. A Regime-Switching (RS) Framework for Formulating Multi-Phase Linear and Nonlinear Growth Curve Models, Sy-Miin Chow, Dongjun You, and Tracy Clouthier. About the Editors.
-
Paperback978-1-64802-222-7
Web price: $45.04 (Reg. 52.99)
-
Hardcover978-1-64802-223-4
Web price: $80.74 (Reg. 94.99)
- eBook978-1-64802-224-1
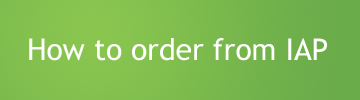
- EDU030000 - EDUCATION: Testing & Measurement
- COM021030 - COMPUTERS: Data Science: Data Analytics
- COM062000 - COMPUTERS: Data Science: Data Modeling & Design
-
Application of Artificial Intelligence to Assessment
-
Data Analytics and Psychometrics Informing Assessment Practices
-
Enhancing Effective Instruction and Learning Using Assessment Data
-
Machine Learning, Natural Language Processing, and Psychometrics
-
Technology Enhanced Innovative Assessment Development, Modeling, and Scoring From an Interdisciplinary Perspective
-
Test Fairness in the New Generation of Large‐Scale Assessment
-
The Next Generation of Testing Common Core Standards, Smarter‐Balanced, PARCC, and the Nationwide Testing Movement